CovidDeep: SARS-CoV-2/COVID-19 Test Based on Wearable Medical Sensors and Efficient Neural Networks
Shayan Hassantabar, Novati Stefano, Vishweshwar Ghanakota, Alessandra Ferrari, Gregory N. Nicola, Raffaele Bruno, Ignazio R. Marino, Niraj K. Jha
The novel coronavirus (SARS-CoV-2) has led to a pandemic. Due to its highly contagious nature, it has spread rapidly, resulting in major disruption to public health. In addition, it has also had a severe negative impact on the world economy. As a result, it is widely recognized now that widespread testing is key to containing the spread of the disease and opening up the economy. However, the current testing regime has been unable to keep up with testing demands. Hence, there is a need for an alternative approach for repeated large-scale testing of COVID-19. The emergence of wearable medical sensors (WMSs) and novel machine learning methods, such as deep neural networks (DNNs), points to a promising approach to address this challenge. WMSs enable continuous and user-transparent monitoring of the physiological signals. However, disease detection based on WMSs/DNNs and their deployment on resource-constrained edge devices remain challenging problems. In this work, we propose CovidDeep, a framework that combines efficient DNNs with commercially available WMSs for pervasive testing of the coronavirus. We collected data from 87 individuals, spanning four cohorts including healthy, asymptomatic (but SARS-CoV-2-positive) as well as moderately and severely symptomatic COVID-19 patients. We trained DNNs on various subsets of the features extracted from six WMS and questionnaire categories to perform ablation studies to determine which subsets are most efficacious in terms of test accuracy for a four-way classification. The highest test accuracy obtained was 99.6%. Since different WMS subsets may be more accessible (in terms of cost, availability, etc.) to different sets of people, we hope these DNN models will provide users with ample flexibility. The resultant DNNs can be easily deployed on edge devices, e.g., smartwatch or smartphone, which also has the benefit of preserving patient privacy.
INTRODUCTION
SARS-COV-2, also known as novel coronavirus, emerged in China and soon after spread across the globe. The World Health Organization (WHO) named the resultant disease COVID-19. COVID-19 was declared a pandemic on March 11, 2020 [1]. In its early stages, the symptoms of COVID-19 include fever, cough, fatigue, and myalgia. However, in more serious cases, it can lead to shortness of breath, pneumonia, severe acute respiratory disorder, and heart problems, and may lead to death [2]. It is of paramount importance to detect which individuals are infected at as early a stage as possible in order to limit the spread of
disease through quarantine and contact tracing. In response to COVID-19, governments around the world have issued social distancing and self-isolation orders. This has led to a significant increase in unemployment across diverse economic sectors. As a result, COVID-19 has triggered an economic recession in a large number of countries [3].
Reverse Transcription-Polymerase Chain Reaction (RTPCR) is currently the gold standard for SARS-CoV-2 detection [4]. This test is based on viral nucleic acid detection in sputum or nasopharyngeal swab. Although it has high specificity, it has several drawbacks. The RT-PCR test is invasive and uncomfortable, and non-reusable testing kits have led to significant supply chain deficiencies. SARS-CoV-2 infection can also be assessed with an antibody test [5]. However, antibody titers are only detectable from the second week of illness onwards and persist for an uncertain length of time. The antibody test is also invasive, requiring venipuncture which, in combination with a several-day processing time, makes it less ideal for rapid mass screening. In the current economic and social situation, there is a great need for an alternative SARS-CoV-2/COVID-19 detection method that is easily accessible to the public for repeated testing with high accuracy.
To address the above issues, researchers have begun to explore the use of artificial intelligence (AI) algorithms to detect COVID-19 [6]. Initial work concentrated on CT scans and X-ray images [4], [7]–[21]. A survey of such datasets can be found in [22], [23]. These methods often rely on transfer learning of a convolutional neural network (CNN) architecture, pre-trained on large image datasets, on a smaller COVID-19 image dataset. However, such an imagebased AI approach faces several challenges that include lack of large datasets and inapplicability outside the clinic or hospital. In addition, other work [24] shows that it is difficult to distinguish COVID-19 pneumonia from influenza virus pneumonia in a clinical setting using CT scans. Thus, the work in this area is not mature yet.
CORD-19 [25] is an assembly of 59000 scholarly articles on COVID-19. It can be used with natural language processing methods to distill useful information on COVID-19-related topics.
AI4COVID-19 [26] performs a preliminary diagnosis of COVID-19 through cough sample recordings with a smartphone application. However, since coughing is a common symptom of two dozen non-COVID-19 medical conditions, this is an extremely difficult task. Nonetheless, AI4COVID-19 shows promising results and opens the door for COVID-19 diagnosis through a smartphone.
The emergence of wearable medical sensors (WMSs) offers a promising way to tackle these challenges. WMSs can continuously sense physiological signals throughout the day [27]. Hence, they enable constant monitoring of the user’s health status. Training AI algorithms with data produced by WMSs can enable pervasive health condition tracking and disease onset detection [28]. This approach exploits the knowledge distillation capability of machine learning algorithms to directly extract information from physiological signals. Thus, it is not limited to disease detection in the clinical scenarios.
We propose a framework called CovidDeep for daily detection of SARS-CoV-2/COVID-19 based on off-the-shelf WMSs and compact deep neural networks (DNNs). It bypasses manual feature engineering and directly distills information from the raw signals captured by available WMSs. It addresses the problem posed by small COVID-19 datasets by relying on intelligent synthetic data generation from the same probability distribution as the training data [29]. These synthetic data are used to pre-train the DNN architecture in order to impose a prior on the network weights. To cut down on the computation and storage costs of the model without any loss in accuracy, CovidDeep leverages the grow-and-prune DNN synthesis paradigm [30], [31]. This not only improves accuracy, but also shrinks model size and reduces the computation costs of the inference process.
The major contributions of this article are as follows:
- We propose CovidDeep, an easy-to-use, accurate, and pervasive SARS-CoV-2/COVID-19 detection framework. It combines features extracted from physiological signals using WMSs and simple-to-answer questions in a smartphone application-based questionnaire with efficient DNNs.
- It uses an intelligent synthetic data generation module to obtain a synthetic dataset [29], labeled by decision rules. The synthetic dataset is used to pre-train the weights of the DNN architecture.
- It uses a grow-and-prune DNN synthesis paradigm that learns both an efficient architecture and weights of the DNN at the same time [30], [31].
- It provides a solution to the daily SARS-CoV-2/COVID-19 detection problem. It captures all the required physiological signals non-invasively through comfortably-worn WMSs that are commercially available.
The rest of the article is organized as follows. Section 2 reviews background material. Section 3 describes the CovidDeep framework. Section 4 provides implementation details. Section 5 presents experimental results. Section 6 provides a short discussion on CovidDeep and possible directions for future research. Finally, Section 7 concludes the article.
[...]
REFERENCES
[1] World Health Organization and others, “Coronavirus disease 2019 (COVID-19): Situation report, 72,” 2020. [Online]. Available: https://apps.who.int/iris/bitstream/handle/10665/ 331685/nCoVsitrep01Apr2020-eng.pdf
[2] E. Mahase, “Coronavirus: COVID-19 has killed more people than SARS and MERS combined, despite lower case fatality rate,” British Medical Journal, vol. 368, 2020. [Online]. Available: https://www.bmj.com/content/368/bmj.m641
[3] M. Nicola, Z. Alsafi, C. Sohrabi, A. Kerwan, A. Al-Jabir, C. Iosifidis, M. Agha, and R. Agha, “The socio-economic implications of the coronavirus and COVID-19 pandemic: A review,” Int. J. Surgery, 2020.
[4] C. Butt, J. Gill, D. Chun, and B. A. Babu, “Deep learning system to screen coronavirus disease 2019 pneumonia,” Applied Intelligence, p. 1, 2020.
[5] K. Dheda, S. Jaumdally, M. Davids, J.-W. Chang, P. Gina, A. Pooran, E. Makambwa, A. Esmail, E. Vardas, and W. Preiser, “Diagnosis of COVID-19: Considerations, controversies and challenges in South Africa,” Wits Journal of Clinical Medicine, vol. 2, no. SI, p. 3, 2020.
[6] J. Bullock, K. H. Pham, C. S. N. Lam, M. Luengo-Oroz et al., “Mapping the landscape of artificial intelligence applications against COVID-19,” arXiv preprint arXiv:2003.11336, 2020.
[7] M. Farooq and A. Hafeez, “COVID-ResNet: A deep learning framework for screening of COVID-19 from radiographs,” arXiv preprint arXiv:2003.14395, 2020.
[8] L. Wang and A. Wong, “COVID-Net: A tailored deep convolutional neural network design for detection of COVID-19 cases from chest radiography images,” arXiv preprint arXiv:2003.09871, 2020.
[9] “SIRM COVID-19 Database,” https://www:sirm:org/category/senza-categoria/covid-19/.
[10] “Diagnosi radiologica e prevenzione della diffusione di COVID-19 nei dipartimenti di radiologia.” [Online]. Available: https://www.sirm.org/wp-content/uploads/2020/03/SIRM-Covid-19.pdf
[11] J. Zhang, Y. Xie, Y. Li, C. Shen, and Y. Xia, “COVID-19 screening on chest X-ray images using deep learning based anomaly detection,”arXiv preprint arXiv:2003.12338, 2020.
[12] A. Narin, C. Kaya, and Z. Pamuk, “Automatic detection of coronavirus disease (COVID-19) using X-ray images and deep convolutional neural networks,” arXiv preprint arXiv:2003.10849, 2020.
[13] A. Abbas, M. M. Abdelsamea, and M. M. Gaber, “Classification of COVID-19 in chest X-ray images using DeTraC deep convolutional neural network,” arXiv preprint arXiv:2003.13815, 2020.
[14] L. O. Hall, R. Paul, D. B. Goldgof, and G. M. Goldgof, “Finding COVID-19 from chest X-rays using deep learning on a small dataset,” arXiv preprint arXiv:2004.02060, 2020.
[15] P. K. Sethy and S. K. Behera, “Detection of coronavirus disease (COVID-19) based on deep features,” Preprints, vol. 2020030300, p. 2020, 2020.
[16] L. Li, L. Qin, Z. Xu, Y. Yin, X. Wang, B. Kong, J. Bai, Y. Lu, Z. Fang, Q. Song et al., “Artificial intelligence distinguishes COVID-19 from community acquired pneumonia on chest CT,” Radiology, p. 200905, 2020.
[17] O. Gozes, M. Frid-Adar, H. Greenspan, P. D. Browning, H. Zhang, W. Ji, A. Bernheim, and E. Siegel, “Rapid AI development cycle for the coronavirus (COVID-19) pandemic: Initial results for automated detection & patient monitoring using deep learning CT image analysis,” arXiv preprint arXiv:2003.05037, 2020.
[18] I. D. Apostolopoulos and T. A. Mpesiana, “COVID-19: Automatic detection from X-ray images utilizing transfer learning with convolutional neural networks,” Physical and Engineering Sciences in Medicine, p. 1, 2020.
[19] S. Wang, Y. Zha, W. Li, Q. Wu, X. Li, M. Niu, M. Wang, X. Qiu, H. Li, H. Yu et al., “A fully automatic deep learning system for COVID-19 diagnostic and prognostic analysis,” medRxiv, 2020.
[20] P. Afshar, S. Heidarian, F. Naderkhani, A. Oikonomou, K. N. Plataniotis, and A. Mohammadi, “COVID-CAPS: A capsule networkbased framework for identification of COVID-19 cases from X-ray images,” arXiv preprint arXiv:2004.02696, 2020.
[21] S. Hassantabar, M. Ahmadi, and A. Sharifi, “Diagnosis and detection of infected tissue of COVID-19 patients based on lung Xray image using convolutional neural network approaches,” Chaos, Solitons & Fractals, vol. 140, p. 110170, 2020.
[22] R. Kalkreuth and P. Kaufmann, “COVID-19: A survey on public medical imaging data resources,” arXiv preprint arXiv:2004.04569, 2020.
[23] J. P. Cohen, P. Morrison, and L. Dao, “COVID-19 image data collection,” arXiv preprint arXiv:2003.11597, 2020.
[24] L. Lin, G. Fu, S. Chen, and J. Tao, “CT manifestation of coronavirus disease (COVID-19) pneumonia and influenza virus pneumonia: A comparative study,” American J. Roentgenology, pp. 1–9, 2020.
[25] “COVID-19 Open Research Dataset (CORD-19),” https://pages:semanticscholar:org/Coronavirus-research.
[26] A. Imran, I. Posokhova, H. N. Qureshi, U. Masood, S. Riaz, K. Ali, C. N. John, and M. Nabeel, “AI4COVID-19: AI enabled preliminary diagnosis for COVID-19 from cough samples via an app,” arXiv preprint arXiv:2004.01275, 2020.
[27] H. Yin and N. K. Jha, “A health decision support system for disease diagnosis based on wearable medical sensors and machine learning ensembles,” IEEE Trans. Multi-Scale Computing Systems, vol. 3, no. 4, pp. 228–241, 2017.
[28] H. Yin, B. Mukadam, X. Dai, and N. Jha, “DiabDeep: Pervasive diabetes diagnosis based on wearable medical sensors and efficient neural networks,” IEEE Trans. Emerging Topics in Computing, 2019.
[29] S. Hassantabar, P. Terway, and N. K. Jha, “TUTOR: Training neural networks using decision rules as model priors,” arXiv preprint arXiv:2010.05429, 2020.
[30] X. Dai, H. Yin, and N. K. Jha, “NeST: A neural network synthesis tool based on a grow-and-prune paradigm,” IEEE Trans. Computers, vol. 68, no. 10, pp. 1487–1497, Oct. 2019.
[31] S. Hassantabar, Z.Wang, and N. K. Jha, “SCANN: Synthesis of compact and accurate neural networks,” arXiv preprint arXiv:1904.09090, 2019.
[32] M. Sandler, A. Howard, M. Zhu, A. Zhmoginov, and L.-C. Chen, “MobileNet v2: Inverted residuals and linear bottlenecks,” in Proc. IEEE/CVF Conf. Computer Vision and Pattern Recognition, 2018, pp. 4510–4520.
[33] N. Ma, X. Zhang, H.-T. Zheng, and J. Sun, “ShuffleNet v2: Practical guidelines for efficient CNN architecture design,” arXiv preprint arXiv:1807.11164, vol. 1, 2018.
[34] B.Wu, A.Wan, X. Yue, P. Jin, S. Zhao, N. Golmant, A. Gholaminejad, J. Gonzalez, and K. Keutzer, “Shift: A zero flop, zero parameter alternative to spatial convolutions,” in Proc. IEEE Conf. Computer Vision and Pattern Recognition, 2018, pp. 9127–9135.
[35] A. Wan, X. Dai, P. Zhang, Z. He, Y. Tian, S. Xie, B. Wu, M. Yu, T. Xu, K. Chen et al., “FBNetV2: Differentiable neural architecture search for spatial and channel dimensions,” in Proc. IEEE Conf. Computer Vision and Pattern Recognition, 2020, pp. 12 965–12 974.
[36] X. Dai, P. Zhang, B. Wu, H. Yin, F. Sun, Y. Wang, M. Dukhan, Y. Hu, Y. Wu, Y. Jia, P. Vajda, M. Uyttendaele, and N. K. Jha, “ChamNet: Towards efficient network design through platformaware model adaptation,” in Proc. IEEE Conf. Computer Vision and Pattern Recognition, 2019.
[37] S. Hassantabar, X. Dai, and N. K. Jha, “STEERAGE: Synthesis of neural networks using architecture search and grow-and-prune methods,” arXiv preprint arXiv:1912.05831, 2019.
[38] X. Dai, A. Wan, P. Zhang, B. Wu, Z. He, Z. Wei, K. Chen, Y. Tian, M. Yu, P. Vajda et al., “FBNetV3: Joint architecture-recipe search using neural acquisition function,” arXiv preprint arXiv:2006.02049, 2020.
[39] S. Han, H. Mao, and W. J. Dally, “Deep compression: Compressing deep neural networks with pruning, trained quantization and Huffman coding,” arXiv preprint arXiv:1510.00149, 2015.
[40] S. Han, J. Kang, H. Mao, Y. Hu, X. Li, Y. Li, D. Xie, H. Luo, S. Yao, Y. Wang et al., “ESE: Efficient speech recognition engine with sparse LSTM on FPGA,” in Proc. ACM/SIGDA Int. Symp. Field- Programmable Gate Arrays, 2017, pp. 75–84.
[41] X. Dai, H. Yin, and N. K. Jha, “Grow and prune compact, fast, and accurate LSTMs,” IEEE Trans. Computers, vol. 69, no. 3, pp. 441–452, Mar. 2020.
[42] C. Zhu, S. Han, H. Mao, and W. J. Dally, “Trained ternary quantization,” arXiv preprint arXiv:1612.01064, 2016.
[43] A. Krizhevsky, I. Sutskever, and G. E. Hinton, “Imagenet classification with deep convolutional neural networks,” in Proc. Advances in Neural Information Processing Systems, 2012, pp. 1097–1105.
[44] S. Grossberg, “Nonlinear neural networks: Principles, mechanisms, and architectures,” Neural Networks, vol. 1, no. 1, pp. 17–61, 1988.
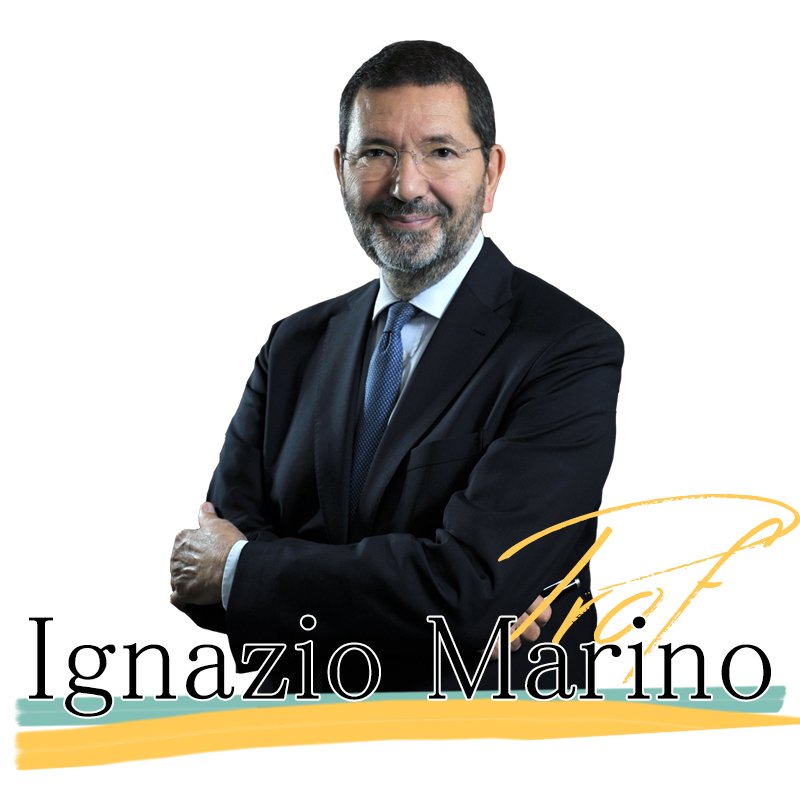